Christophe Garcia
Full Professor at Insa Lyon & LIRIS Laboratory (UMR 5205 CNRS)
Enhancing Face Recognition from Video Sequences using Robust Statistics
SA. Berrani, C. Garcia, France Telecom R&D, 2008
The impact of outliers on the performance of high-dimensional data analysis methods is studied in the context of face recognition. Most of the existing face recognition methods are based on PCA-like methods: faces are projected into a lower dimensional space in which similarity between faces is supposed to be more easily evaluated. These methods are, however, very sensitive to the quality of the face images used in the training and in the recognition phases. Their performance significantly drops when face images are not well centered or taken under variable illumination conditions. In this paper, we study this phenomenon for two face recognition methods, namely PCA and LDA2D, and we propose a filtering process that allows the automatic selection of noisy face images which are responsible for the performance degradation. This process uses two techniques. The first one is based on the recently proposed robust high-dimensional data analysis method called RobPCA. It is specific to the case of recognition from video sequences. The second technique is based on a novel and effective face classification technique. It allows isolating still face images that are not very precisely cropped, not well-centered or in a non-frontal pose. Experiments show that this filtering process significantly improves recognition rates by 10 to 30%.
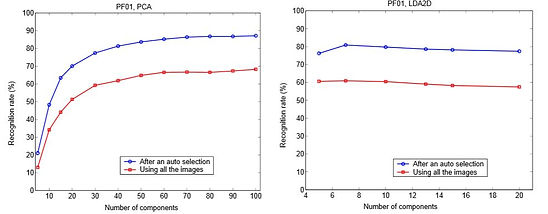
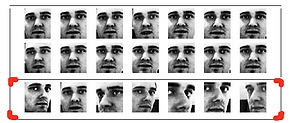
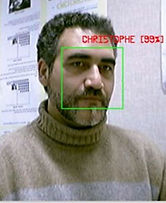